The game is simple. Users create a monster character and profile, start swiping right or left on other monsters’ accounts, chat, and date. I created, Ian, a blue monster with questionable hair and it didn’t take long to match with Octiana, a Disney enthusiast, who eventually got my number. The longer I played, the more the game got to know my “monster preferences” — it showed my most and least recommended profiles and found a pattern and eventually I was left seeing the same monsters time and time again. An algorithm can have unintended consequences since it takes multiple factors into account, such as your early actions on the app. Because of this, potential matches may never be shown, in turn discriminating against races, ethnicities, and sexual orientations. MonsterMatch illustrates this by showing a werewolf swiping “yes” on a zombie, which then swipes “no” on a vampire. From then on, when a new user also swipes “yes” on the zombie, the algorithm will assume the new user also dislikes the vampire, and will then withhold vampire profiles from this user. “Collaborative filtering in dating means that the earliest and most numerous users of the app have outsize influence on the profiles later users see. Some early user says she likes (by swiping right on) some other active dating app user. Then that same early user says she doesn’t like (by swiping left on) a Jewish user’s profile, for whatever reason,” MonsterMatch’s blog outlined. Multiple studies have backed up the games’ speculations about racial bias on dating apps. For example, APA PsycNET found that black people are ten times more likely to contact white people on dating sites than vice versa. And in 2014, OKCupid found that black women and Asian men were likely to be rated lower than other ethnic groups on the site. Although MonsterMatch seems like a bit of fun, this computer game is doing a lot more than just entertaining — it’s working towards educating people on how dating apps really work, and how their swipes may affect their future matches and fuel racial bias.
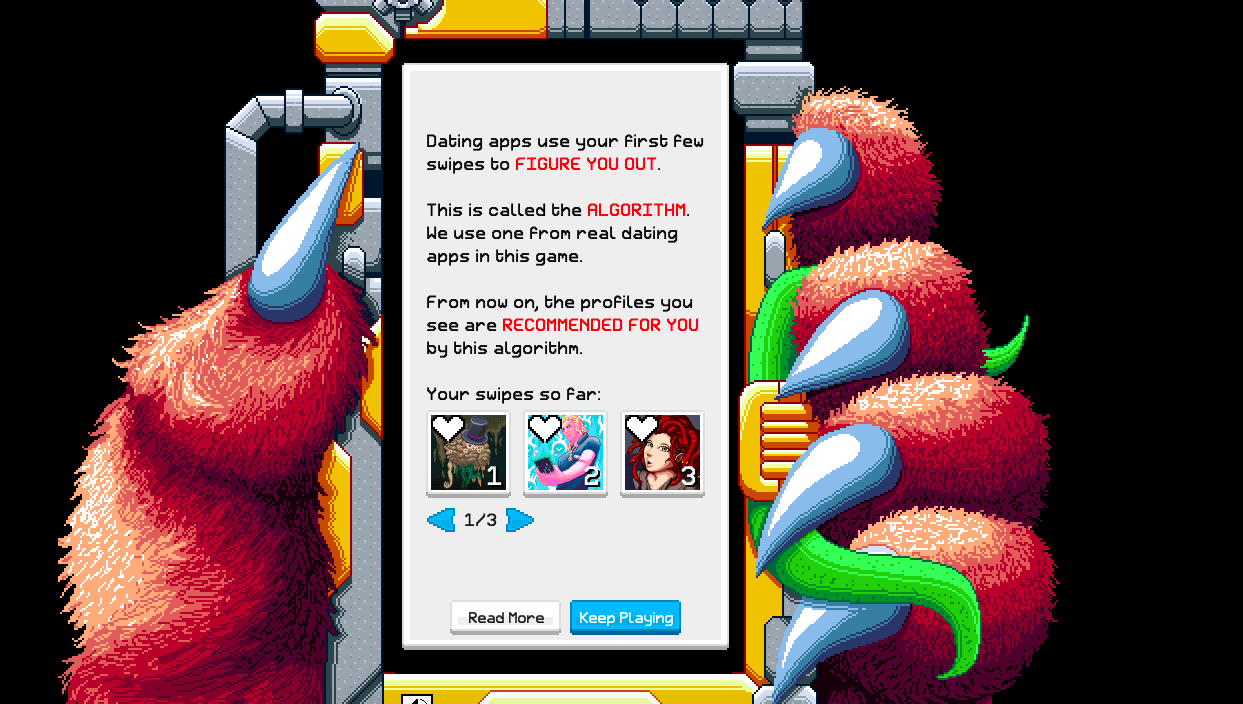